Ivatury and Alambeigi have acquired a grant from UT’s IC2 Institute to develop the tactile sensor and hope that inside 5 years it could possibly be in scientific trials. In the meantime, Ivatury and Marculescu have acquired a Texas Catalyst Grant from UT and Dell Med to pursue their analysis on the usage of AI in colonoscopy.
In each tasks — the visible (Marculescu) and the tactile (Alambeigi) — AI can be doing three issues:
First, it’s studying. Ivatury thinks of the problem when it comes to yard work. “It’s like this huge garden, and we have to take out solely the weeds. What we’re doing is coaching the system with all of the totally different attainable kinds of weeds, totally different yards, telling it: ‘It is a weed; that is grass.’” These “footage” within the analogy are knowledge from two sources: colonoscopy pictures of Ivatury’s at Dell Med, and publicly out there well being knowledge units. “What we’re doing now could be getting footage of actual yards and actual weeds, pulling these weeds and ensuring they’re actually weeds based mostly on pathology,” he says.
“We’re going to make use of that sensor on actual pathology from my surgical specimens, my sufferers.” The range of the sufferers coming by way of the door at Dell Seton (Dell Med’s educating hospital) and UT Well being Austin (Dell Med’s scientific/outpatient arm) places the analysis a lot farther forward. “As a result of our affected person inhabitants at Dell Med is a various inhabitants, we all know not solely that the affected person has this illness course of, we all know in the event that they’re male or feminine, white Caucasian, Hispanic, African American, Asian. We are able to be certain that our enter knowledge set is balanced when it comes to race, gender, age, ethnicity, in addition to illness course of,” says Ivatury.
Second, AI will take what it has realized from actual samples and extrapolate to create a bigger database of AI-generated ones. Consider this as importing lots of of images of yards with and with out weeds, then creating hundreds of different footage that can train the machine to establish them in any attainable situation with regard to angle, lighting and the encompassing topography of the colon, which is difficult to say the least.
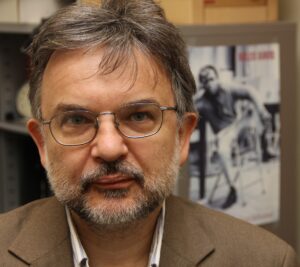
Upon getting sufficient knowledge from actual life — both visible knowledge from the digital camera or from the tactile sensor, AI can then make up “phantom knowledge.” When you’ve got 50 numerous sufferers, it could actually create phantom sufferers with most cancers, Crohn’s illness, and so forth. In our lawns-and-weeds analogy, Marculescu says artificial knowledge technology is like creating a number of lawns in numerous contexts equivalent to totally different shaded areas or viewing angles. “The thought can be for a few of them to be laborious to catch,” he provides.
“I don’t want to do that for 10,000 sufferers to make sure we now have all of the steadiness,” says Ivatury. “AI can extrapolate from the info we put in to create a various group of ‘phantom’ sufferers that in flip are used to coach the algorithm.” As soon as he will get sufficient knowledge, he can use the artificial knowledge technology to create as many yards as wanted with each mixture of weeds, and a few yards with no weeds, in order that by the point it will get to an actual affected person, the algorithm has seen most if not all mixtures. And because it continues to see extra sufferers, it would collect extra knowledge and proceed to enhance.
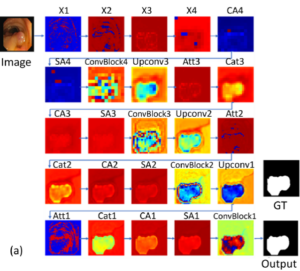
Marculescu says artificial knowledge technology, used to compensate for a shortage of information, can be “massively impactful if accomplished correctly.” He likens artificial knowledge technology to taking observe assessments within the hope that whenever you take the actual take a look at, you’ll do higher on it. Getting a colonoscopy is a traumatic, sophisticated process, he says, so persons are not going to voluntarily line as much as have them for the sake of making a bigger AI database. Shortage of information within the medical area will at all times be an issue, he says, as a result of it’s often invasive.
On the whole, the bigger the pattern dimension the extra seemingly the algorithm is to detect a polyp. However as with most purposes of AI, customers should be on guard in opposition to bias. “Bias is a large subject within the medical area,” Marculescu says. How can we assure that knowledge and machine studying are usually not biasing one gender over one other or one race or ethnicity over one other? And bias just isn’t solely and even primarily a matter of race or gender: There are various kinds of polyps, two of that are quite common. If we’re utilizing a small pattern dimension to coach the algorithm, then it would change into biased towards the 2 widespread varieties making it extra more likely to miss the rarer varieties.
Extrapolation from a various set of real-life instances is a case of utilizing AI to beat an issue inherent to AI — bias. If they will use AI to create artificial pictures which might be as reasonable as precise pictures, then these can be utilized to show the algorithm in a extra fulsome method, thus mitigating that bias. Alambeigi says, “We’re proving that artificial pictures are nearly as good as actual pictures.”
Lastly, AI will help within the process. AI throughout a colonoscopy will management for the variations within the usually sophisticated contours of even a wholesome colon, for the lighting, and, in situations when the colon just isn’t fully clear, for obscuring.
When scientists began benchmarking AI’s potential to categorise objects, the error charge was astronomical, 30-40%, in keeping with Marculescu. However by 2014, AI had surpassed people’ imaginative and prescient, when the error grew to become lower than 3.5%. As exceptional as that is, Marculescu says, “The fact is that even that end result must be taken with a grain of salt as a result of coping with sufferers just isn’t the identical factor as coping with pictures of cats and canine and vehicles and automobiles and pictures which might be effectively lit.”
The distinction between sick and wholesome tissue might be extraordinarily refined. Because the new AI-assisted imaging is designed to work on the pixel degree (the tiniest unit of a video show), it ought to assist endoscopists through the process. “In actual time, it’s going to work within the background whilst you’re having your colonoscopy as a result of if it doesn’t, then what’s the purpose of doing it?” asks Ivatury. “We want to have the ability to say, ‘Hey, look over there, there’s one thing suspicious. You must test that out, you need to see the way it feels and possibly take it out.’”
A part of Alambeigi’s pleasure about AI is that it could actually assist cut back the subjective nature of exams in order that much less skilled endoscopists have the identical “resolution cowl.” One other use of AI is that it could actually use the info to create extra reasonable simulators (assume flight simulators) for physician coaching. Lastly, by way of AI language fashions, docs can have dialogues with the system by prompting it with voice instructions to regulate the digital camera view equivalent to zooming in and zooming out and conceivably even asking questions on what the system is seeing.
Furthermore, the implications of this work under no circumstances cease at partitions of the colon however are transferable to innumerable different areas of drugs the place visible data is essential. In relation to surgical procedure, whether or not dental implants or mind surgical procedure, surgeons almost at all times use an help to assist them see extra carefully. AI, says Marculescu, is just yet one more such instrument.